Haidar Khan
Research Scientist (Meta, SDAIA, Amazon). CTO and co-founder (ai.astrolabe). Partner (KhanTeT).
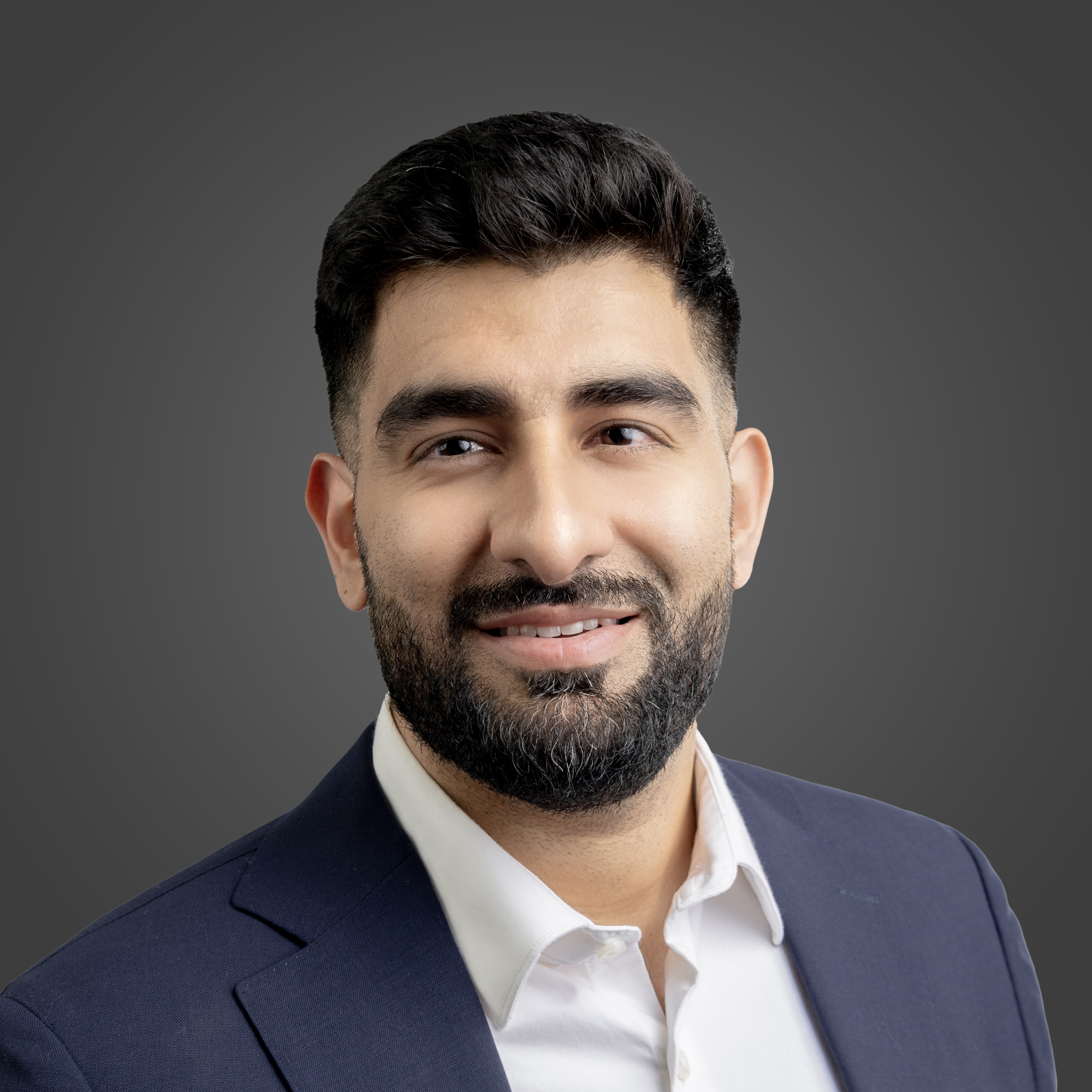
Kingston, New York 12401
My research interests cover a broad range of topics in NLP and machine learning. My recent work is mainly focused around understanding how to build efficient (small/fast) models efficiently (without breaking the compute bank) for NLP tasks. I am also excited about ZeroSumEval, a project we started to scale evaluation of LLMs with compute and capabilties.
I’ve had the privilege of pursuing this work with amazing colleages at industry and academia. Most recently, I was granted Premium Residency for special talent in Saudi Arabia and spent a year with the National Center of AI (NCAI) at the Saudi Data and AI Authority (SDAIA) as a research scientist. Our team published research (1, 2, 3) on large language models (LLM) and built ALLaM, the best LLM for Arabic and English (at the time).
Prior to that, I was a senior applied scientist at Amazon Alexa AI conducting research in NLP topics (semantic parsing, efficient modeling) and helped build Alexa Teacher Models, Amazon’s precursor to Amazon General Intelligence.
I completed my PhD with the Data Science Research Center (DSRC) at Rensselaer Polytechnic Institute (RPI). My work with Prof. Bülent Yener focused on developing methods specially designed for classifying, predicting, and analyzing medical data. I was fortunate to work closely with a team of doctors from the Mount Sinai Hospital Epilepsy Center and witness the impact of my work on patient care.
The rest of my time is dedicated to my family and pursuing my other passions; learning (human) langauges (4 so far), Quran and Arabic poetry, riding horses (western), and hunting.
news
Sep 30, 2024 | We founded ai.astrolabe |
---|---|
Aug 21, 2023 | I joined the National Center for Artificial Intelligence in Riyadh, Saudi Arabia as a visiting scientist. |
latest posts
Oct 08, 2024 | A journey into NLP |
---|
selected publications
- Learning filter widths of spectral decompositions with waveletsAdvances in Neural Information Processing Systems, 2018
- Don’t Parse, Insert: Multilingual Semantic Parsing with Insertion Based DecodingarXiv preprint arXiv:2010.03714, 2020
- Compressing Transformer-Based Semantic Parsing Models using Compositional Code EmbeddingsarXiv preprint arXiv:2010.05002, 2020
- RescoreBERT: Discriminative Speech Recognition Rescoring With BertIn ICASSP 2022-2022 IEEE International Conference on Acoustics, Speech and Signal Processing (ICASSP), 2022
- Alexa teacher model: Pretraining and distilling multi-billion-parameter encoders for natural language understanding systemsIn Proceedings of the 28th ACM SIGKDD Conference on Knowledge Discovery and Data Mining, 2022
- AlexaTM 20B: Few-Shot Learning Using a Large-Scale Multilingual Seq2Seq ModelarXiv preprint arXiv:2208.01448, 2022
- When Benchmarks are Targets: Revealing the Sensitivity of Large Language Model LeaderboardsarXiv preprint arXiv:2402.01781, 2024
- A Systematic Survey and Critical Review on Evaluating Large Language Models: Challenges, Limitations, and RecommendationsarXiv preprint arXiv:2407.04069, 2024
-